Modern AI models possess hidden capabilities that emerge suddenly and consistently during training, but these abilities remain concealed until prompted in specific ways, according to new research from Harvard and the University of Michigan.
The study, which analyzed how AI systems learn concepts like color and size, revealed that models often master these skills far earlier than standard tests suggest—a finding with major implications for AI safety and development.
“Our results demonstrate that measuring an AI system’s capabilities is more complex than previously thought,” the research paper says. “A model might appear incompetent when given standard prompts while actually possessing sophisticated abilities that only emerge under specific conditions.”
This advancement joins a growing body of research aimed at demystifying how AI models develop capabilities.
Anthropic researchers unveiled “dictionary learning,” a technique that mapped millions of neural connections within their Claude language model to specific concepts the AI understands, Decrypt reported earlier this year.
While approaches differ, these studies share a common goal: bringing transparency to what has primarily been considered AI’s “black box” of learning.
“We found millions of features which appear to correspond to interpretable concepts ranging from concrete objects like people, countries, and famous buildings to abstract ideas like emotions, writing styles, and reasoning steps,” Anthropic said in its research paper.
The researchers conducted extensive experiments using diffusion models—the most popular architecture for generative AI. While tracking how these models learned to manipulate basic concepts, they discovered a consistent pattern: capabilities emerged in distinct phases, with a sharp transition point marking when the model acquired new abilities.
Models showed mastery of concepts up to 2,000 training steps earlier than standard testing could detect. Strong concepts emerged around 6,000 steps, while weaker ones appeared around 20,000 steps.
When researchers adjusted the “concept signal,” the clarity with which ideas were presented in training data.
They found direct correlations with learning speed. Alternative prompting methods could reliably extract hidden capabilities long before they appeared in standard tests.
This phenomenon of “hidden emergence” has significant implications for AI safety and evaluation. Traditional benchmarks may dramatically underestimate what models can actually do, potentially missing both beneficial and concerning capabilities.
Perhaps most intriguingly, the team discovered multiple ways to access these hidden capabilities. Using techniques they termed “linear latent intervention” and “overprompting,” researchers could reliably extract sophisticated behaviors from models long before these abilities appeared in standard tests.
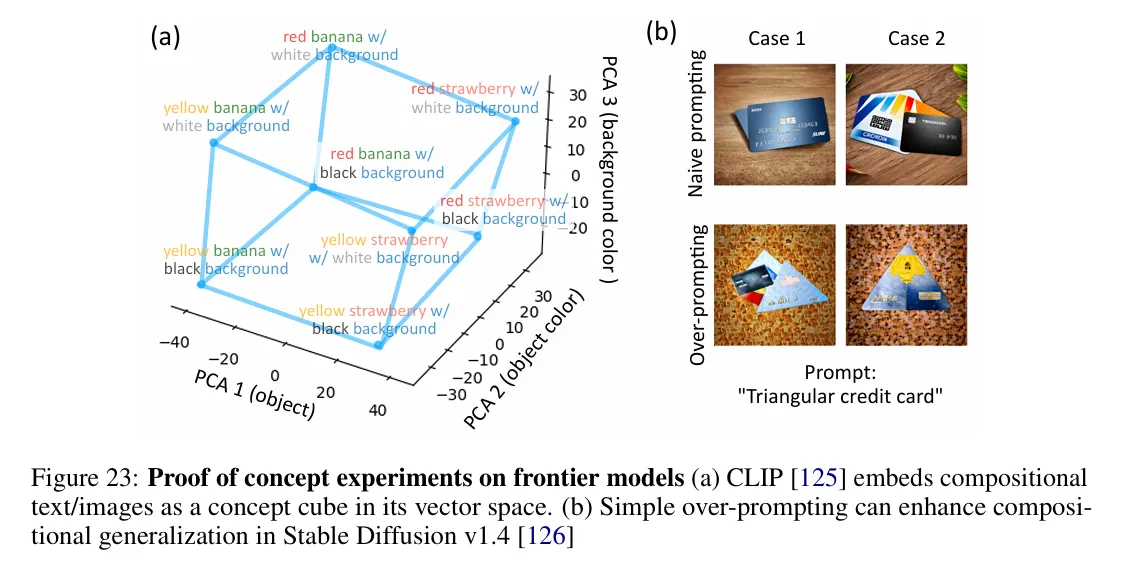
In another case, researchers found that AI models learned to manipulate complex features like gender presentation and facial expressions before they could reliably demonstrate these abilities through standard prompts.
For example, models could accurately generate “smiling women” or “men with hats” individually before they could combine these features—yet detailed analysis showed they had mastered the combination much earlier. They simply couldn’t express it through conventional prompting.
The sudden emergence of capabilities observed in this study might initially seem similar to grokking—where models abruptly demonstrate perfect test performance after extended training—but there are key differences.
While grokking occurs after a training plateau and involves the gradual refinement of representations on the same data distribution, this research shows capabilities emerging during active learning and involving out-of-distribution generalization.
The authors found sharp transitions in the model’s ability to manipulate concepts in novel ways, suggesting discrete phase changes rather than the gradual representation improvements seen in grokking.
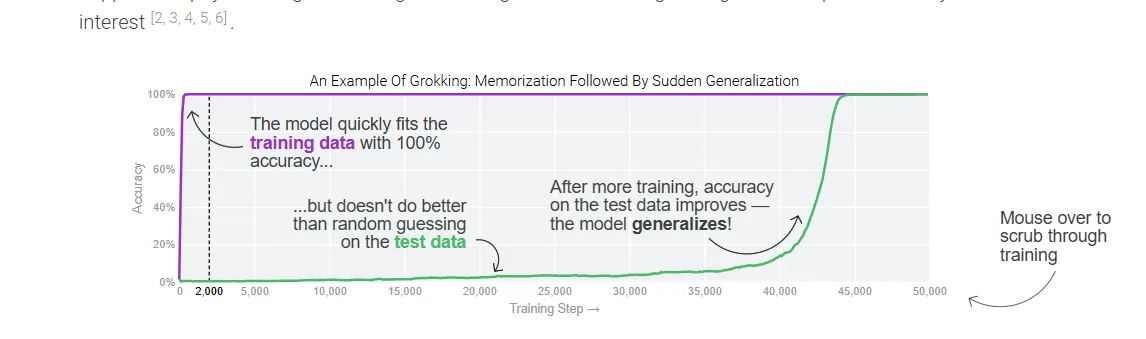
In other words, it seems AI models internalize concepts way earlier than we thought, they are just not able to show their skills—kind of how some people may understand a movie in a foreign language but still struggle to properly speak it.
For the AI industry, this is a double-edged sword. The presence of hidden capabilities indicates models might be more potent than previously thought. Still, it also proves how difficult it is to understand and control what they can do fully.
Companies developing large language models and image generators may need to revise their testing protocols.
Traditional benchmarks, while still valuable, may need to be supplemented with more sophisticated evaluation methods that can detect hidden capabilities.
Edited by Sebastian Sinclair
Generally Intelligent Newsletter
A weekly AI journey narrated by Gen, a generative AI model.
Source link
Jose Antonio Lanz
https://decrypt.co/292892/ai-models-secretly-learn-capabilities-long-before-they-show-them-researchers-find
2024-11-24 15:01:02