A new technique could put AI models on a strict energy diet, potentially cutting power consumption by up to 95% without compromising quality.
Researchers at BitEnergy AI, Inc. have developed Linear-Complexity Multiplication (L-Mul), a method that replaces energy-intensive floating-point multiplications with simpler integer additions in AI computations.
For those unfamiliar with the term, floating-point is a mathematical shorthand that allows computers to handle very large and very small numbers efficiently by adjusting the placement of the decimal point. You can think of it like scientific notation, in binary. They are essential for many calculations in AI models, but they require a lot of energy and computing power. The bigger the number, the better the model is—and the more computing power it requires. Fp32 is generally a full precision model, with developers reducing precision to fp16, fp8, and even fp4, so their models can run on local hardware.
AI’s voracious appetite for electricity has become a growing concern. ChatGPT alone gobbles up 564 MWh daily—enough to power 18,000 American homes. The overall AI industry is expected to consume 85-134 TWh annually by 2027, roughly the same as Bitcoin mining operations, according to estimations shared by the Cambridge Centre for Alternative Finance.
L-Mul tackles the AI energy problem head-on by reimagining how AI models handle calculations. Instead of complex floating-point multiplications, L-Mul approximates these operations using integer additions. So, for example, instead of multiplying 123.45 by 67.89, L-Mul breaks it down into smaller, easier steps using addition. This makes the calculations faster and uses less energy, while still maintaining accuracy.
The results seem promising. “Applying the L-Mul operation in tensor processing hardware can potentially reduce 95% energy cost by element wise floating point tensor multiplications and 80% energy cost of dot products,” the researchers claim. Without getting overly complicated, what that means is simply this: If a model used this technique, it would require 95% less energy to think, and 80% less energy to come up with new ideas, according to this research.
The algorithm’s impact extends beyond energy savings. L-Mul outperforms current 8-bit standards in some cases, achieving higher precision while using significantly less bit-level computation. Tests across natural language processing, vision tasks, and symbolic reasoning showed an average performance drop of just 0.07%—a negligible tradeoff for the potential energy savings.
Transformer-based models, the backbone of large language models like GPT, could benefit greatly from L-Mul. The algorithm seamlessly integrates into the attention mechanism, a computationally intensive part of these models. Tests on popular models such as Llama, Mistral, and Gemma even revealed some accuracy gain on certain vision tasks.
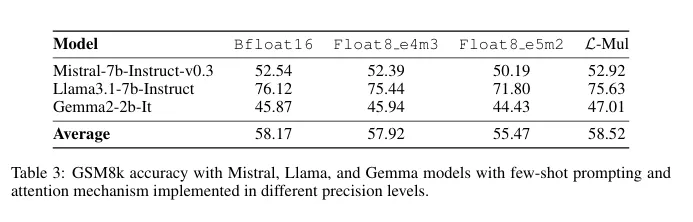
At an operational level, L-Mul’s advantages become even clearer. The research shows that multiplying two float8 numbers (the way AI models would operate today) requires 325 operations, while L-Mul uses only 157—less than half. “To summarize the error and complexity analysis, L-Mul is both more efficient and more accurate than fp8 multiplication,” the study concludes.
But nothing is perfect and this technique has a major achilles heel: It requires a special type of hardware, so the current hardware isn’t optimized to take full advantage of it.
Plans for specialized hardware that natively supports L-Mul calculations may be already in motion. “To unlock the full potential of our proposed method, we will implement the L-Mul and L-Matmul kernel algorithms on hardware level and develop programming APIs for high-level model design,” the researchers say. This could potentially lead to a new generation of AI models that are fast, accurate, and super cheap—making energy-efficient AI a real possibility.
Generally Intelligent Newsletter
A weekly AI journey narrated by Gen, a generative AI model.
Source link
Jose Antonio Lanz
https://decrypt.co/285154/new-technique-slashes-ai-energy
2024-10-08 18:56:57